The Rise of Human-Led Automation
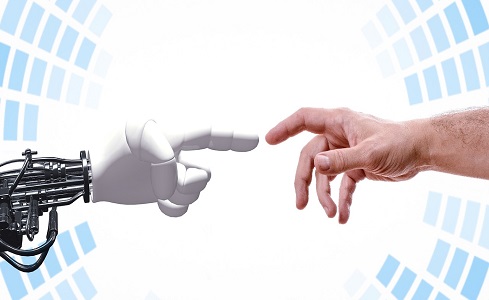
Data is the most crucial useful resource an firm possesses. Knowledge makes it possible for us to make informed choices. It gives important insights into our customers and the encounters we supply. It can help make operational efficiencies that guide to reduced costs and increased margins.
But, right now, we’re drowning in information. We have so a great deal that it is turn out to be complicated to form superior, suitable data we will need from the noise we never. We’re spending a fortune accumulating, handling, and examining data throughout the organization, but we’re not observing the ROI.
Thankfully, automation driven by synthetic intelligence (AI) and machine mastering (ML) is encouraging us get a much better handle on our knowledge. Software package can now look for through huge information sets to discover the applicable info for every purpose. It does not subject if we’re drowning in data — the devices will explain to us what is very good and what is undesirable.
Or so we thought. It’s possible automation isn’t the silver bullet we consider it is.
The Problem of Machines
At the most essential level, automation enlists a machine to conduct rote, repetitive duties extra cheaply and successfully than a human. Whether or not it is a die lower press punching out thousands of identical circles or AI recommending your subsequent movie, the basic principle is the identical.
The electronic age has introduced trivial conveniences like reminders to order far more laundry detergent to lifetime-conserving operations like donor matching. None of this is achievable with out automation. But devices can only get us 90% there. They are excellent at consuming and examining substantial volumes of data but however have difficulties with edge conditions. Guaranteed, we can continue to prepare algorithms to protect much more of these exceptions, but at a particular point, the amount of resources heading into advancement starts off to outweigh the reward.
This potential to quickly and seamlessly apply identified rules and criteria to edge instances is what sets human beings apart from devices. We’re precise thinkers. We can glance at an instance and make a best judgement selection that’s just about invariably appropriate. Equipment are approximators. They appear at the total and come to a decision primarily based on how related use circumstances were being dealt with beforehand, normally delivering very poor effects.
Therein lies the AI paradox: The additional we automate details analytics, the more perform is necessary of individuals to deal with edge situations, provide significant-degree scrutiny, and set that means at the rear of the insights.
The Increase of Human-Led Automation
To push AI in a sensible, productive, and ethical way, enterprises require to allow devices do what they excel at but make positive individuals are there to offer supervision. Centered on explainable AI, the idea that final results will need to be understood and spelled out by people, this is a arms-on, constant cycle that requires involvement in each phase of AI from trouble definition and advancement to ongoing knowledge governance.
Below are a few factors for putting the human contact back again into AI-run solutions:
1. Established corporate values
AI is only as good as the details you feed into it. If existing procedures are implicitly biased, then any algorithm centered on these historical precedents will carry people biases more than to automated processes. Enterprises very first must determine the values they treatment about, make certain human compliance, and then apply all those values to automatic processes.
2. Place people at the supply of instructing
In machine-educated discovering, AI makes and trains an algorithm without human intervention. Equipment really do not have ethics or morals and just can’t make judgement calls. All they know is what’s been taught to them, and, like a activity of phone, these lessons have a tendency to be watered down the even more they get from a human. Creating humans teach algorithms is a get-earn. Human beings can identify and instruct edge cases for equipment whilst equipment offload considerably of the manual, cumbersome jobs.
3. Guarantee human-led governance
AI types want to be continually monitored, calculated, and recalibrated. Still left alone, these types can unintentionally change based mostly on exterior things. Termed drift, these shifts can guide to unintended and undesired benefits. In the same way, moral AI, a component of Explainable AI, guarantees that equipment run less than a process or moral concepts defined by developers. If designs drift far adequate, they can drop their means to act as meant. Although checking drift can be accomplished by devices, any issues that come up require to be escalated to a human who can make a judgement call whether to intervene. Subsequent schooling should also be dealt with by people, ensuring that the algorithm is recalibrated to return exceptional benefits. It is clear that humans—with the correct topic subject expertise—are the ideal judges of product drift. Only they, not machines, have the substantial-level encounter, cognitive skill, and understanding of vital nuances to make these judgement decisions.
Retaining Machines Sincere Calls for a Human Touch
AI has the energy to rework the way we function, are living and participate in, but we still will need humans to instill typical sense and supervision that only folks can present. Putting the human touch back again into automation calls for a determination that begins with defining and enshrining corporate values and continues by way of algorithm enhancement, teaching, and ongoing governance. Machines will one working day take on a a great deal much larger position in our each day life, but we nonetheless want people to preserve them genuine.